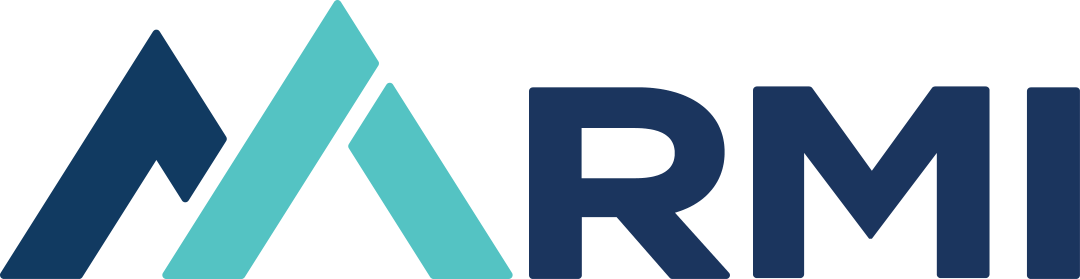
Add targets for production, using the market share approach
Source:R/target_market_share.R
target_market_share.Rd
This function calculates the portfolio-level production targets, as calculated using the market share approach applied to each relevant climate production forecast.
Usage
target_market_share(
data,
abcd,
scenario,
region_isos = r2dii.data::region_isos,
use_credit_limit = FALSE,
by_company = FALSE,
weight_production = TRUE,
increasing_or_decreasing = r2dii.data::increasing_or_decreasing
)
Arguments
- data
A "data.frame" like the output of
r2dii.match::prioritize
.- abcd
An asset level data frame like r2dii.data::abcd_demo.
- scenario
A scenario data frame like r2dii.data::scenario_demo_2020.
- region_isos
A data frame like r2dii.data::region_isos (default).
- use_credit_limit
Logical vector of length 1.
FALSE
defaults to using the columnloan_size_outstanding
. Set toTRUE
to use the columnloan_size_credit_limit
instead.- by_company
Logical vector of length 1.
FALSE
defaults to outputtingproduction_value
at the portfolio-level. Set toTRUE
to outputproduction_value
at the company-level.- weight_production
Logical vector of length 1.
TRUE
defaults to outputting production, weighted by relative loan-size. Set toFALSE
to output the unweighted production values.- increasing_or_decreasing
A data frame like r2dii.data::increasing_or_decreasing.
Value
A tibble including the summarized columns metric
, production
,
technology_share
, percentage_of_initial_production_by_scope
and
scope
. If by_company = TRUE
, the output will also have the column
name_abcd
.
See also
Other functions to calculate scenario targets:
target_sda()
Examples
library(r2dii.data)
library(r2dii.match)
loanbook <- head(loanbook_demo, 100)
abcd <- head(abcd_demo, 100)
matched <- loanbook %>%
match_name(abcd) %>%
prioritize()
# Calculate targets at portfolio level
matched %>%
target_market_share(
abcd = abcd,
scenario = scenario_demo_2020,
region_isos = region_isos_demo
)
#> # A tibble: 373 × 10
#> sector technology year region scenario_source metric production
#> <chr> <chr> <int> <chr> <chr> <chr> <dbl>
#> 1 power hydrocap 2020 global demo_2020 projected 16990.
#> 2 power hydrocap 2020 global demo_2020 target_cps 16990.
#> 3 power hydrocap 2020 global demo_2020 target_sds 16990.
#> 4 power hydrocap 2020 global demo_2020 target_sps 16990.
#> 5 power hydrocap 2021 global demo_2020 projected 16743.
#> 6 power hydrocap 2021 global demo_2020 target_cps 17004.
#> 7 power hydrocap 2021 global demo_2020 target_sds 17012.
#> 8 power hydrocap 2021 global demo_2020 target_sps 17005.
#> 9 power hydrocap 2022 global demo_2020 projected 16497.
#> 10 power hydrocap 2022 global demo_2020 target_cps 17018.
#> # ℹ 363 more rows
#> # ℹ 3 more variables: technology_share <dbl>, scope <chr>,
#> # percentage_of_initial_production_by_scope <dbl>
# Calculate targets at company level
matched %>%
target_market_share(
abcd = abcd,
scenario = scenario_demo_2020,
region_isos = region_isos_demo,
by_company = TRUE
)
#> Warning: You've supplied `by_company = TRUE` and `weight_production = TRUE`.
#> This will result in company-level results, weighted by the portfolio
#> loan size, which is rarely useful. Did you mean to set one of these
#> arguments to `FALSE`?
#> # A tibble: 1,408 × 11
#> sector technology year region scenario_source name_abcd metric production
#> <chr> <chr> <int> <chr> <chr> <chr> <chr> <dbl>
#> 1 power hydrocap 2020 global demo_2020 Giordano, G… proje… 16990.
#> 2 power hydrocap 2020 global demo_2020 Giordano, G… targe… 16990.
#> 3 power hydrocap 2020 global demo_2020 Giordano, G… targe… 16990.
#> 4 power hydrocap 2020 global demo_2020 Giordano, G… targe… 16990.
#> 5 power hydrocap 2021 global demo_2020 Giordano, G… proje… 16743.
#> 6 power hydrocap 2021 global demo_2020 Giordano, G… targe… 17004.
#> 7 power hydrocap 2021 global demo_2020 Giordano, G… targe… 17012.
#> 8 power hydrocap 2021 global demo_2020 Giordano, G… targe… 17005.
#> 9 power hydrocap 2022 global demo_2020 Giordano, G… proje… 16497.
#> 10 power hydrocap 2022 global demo_2020 Giordano, G… targe… 17018.
#> # ℹ 1,398 more rows
#> # ℹ 3 more variables: technology_share <dbl>, scope <chr>,
#> # percentage_of_initial_production_by_scope <dbl>
matched %>%
target_market_share(
abcd = abcd,
scenario = scenario_demo_2020,
region_isos = region_isos_demo,
# Calculate unweighted targets
weight_production = FALSE
)
#> # A tibble: 373 × 10
#> sector technology year region scenario_source metric production
#> <chr> <chr> <int> <chr> <chr> <chr> <dbl>
#> 1 power hydrocap 2020 global demo_2020 projected 121032.
#> 2 power hydrocap 2020 global demo_2020 target_cps 121032.
#> 3 power hydrocap 2020 global demo_2020 target_sds 121032.
#> 4 power hydrocap 2020 global demo_2020 target_sps 121032.
#> 5 power hydrocap 2021 global demo_2020 projected 119274.
#> 6 power hydrocap 2021 global demo_2020 target_cps 121129.
#> 7 power hydrocap 2021 global demo_2020 target_sds 121187.
#> 8 power hydrocap 2021 global demo_2020 target_sps 121139.
#> 9 power hydrocap 2022 global demo_2020 projected 117515.
#> 10 power hydrocap 2022 global demo_2020 target_cps 121227.
#> # ℹ 363 more rows
#> # ℹ 3 more variables: technology_share <dbl>, scope <chr>,
#> # percentage_of_initial_production_by_scope <dbl>